Ali Rahnavard
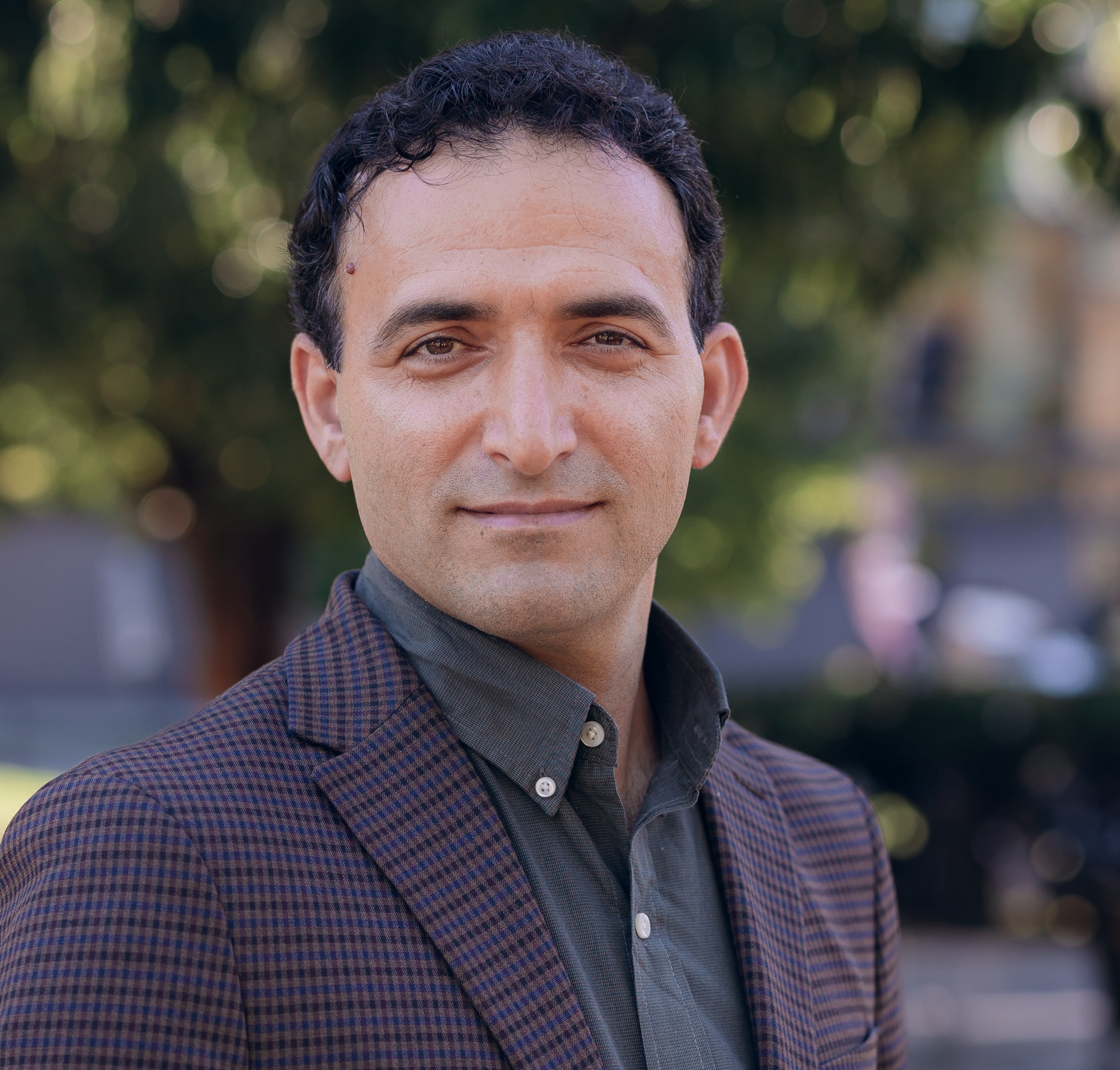
Ali Rahnavard
M.S., Ph.D.
Associate Professor, Director of BS in Health Data Science
School: Milken Institute School of Public Health
Department: Biostatistics and Bioinformatics
Contact:
Ali Rahnavard is an Associate Professor of Biostatistics and Bioinformatics at George Washington University. Dr. Rahnavard is interested in the intersection of the microbiome and metabolome for understanding their interactions in health and disease. Since the metabolome is the interface mediating this interaction, he primarily investigates metabolite and microbiome changes over the course of disease. His lab uses systems-biology-based approaches, applying computational methods to multi-omic data with the goal of generating hypotheses of the underlying processes involved in disease activity. These hypotheses with strong evidence in measured data are suitable for testing in a laboratory and translation into actionable diagnostics and therapeutics.
The Rahnavard lab also develops novel computational methods to investigate how the microbes in the human gut and metabolites interact with each other and with the host during health and disease. As part of this work, Rahnavard lab developed a computational environment for omics data analysis and integration. This framework includes methods for discovering biological patterns in high-dimensional multi-omic datasets and also analyzing metabolite profiles using liquid chromatography tandem mass spectrometry (LC-MS). Using computational techniques, Rahnavard characterized microbial behavior at a deep resolution of strain and function (e.g., how microbial species at the strain level are associated with human body sites) by applying statistical methods to several large cohort-based microbiome studies, including the expanded NIH Human Microbiome Project (HMP1-II) study of the healthy human microbiome.
Rahnavard earned his Ph.D. in computer science, applied statistics, and bioinformatics at New Mexico State University. Rahnavard completed postdoctoral work in the biostatistics department at Harvard T.H. Chan School of Public Health and the Infectious Disease and Microbiome Program at the Broad. Prior to his position with the George Washington University, Rahnavard was a senior computational scientist with the Broad’s Metabolomics Platform. He also holds a master’s degree in computer engineering/software systems from Shiraz University and a bachelor’s degree in computer engineering from Razi University of Kermanshah.
EDUCATION:
Senior Computational Scientist, Metabolomics Platform, The Broad Institute of MIT and Harvard, 2019
Postdoctoral Associate, Department of Biostatistics at Harvard T.H. Chan School of Public Health, 2018
Postdoctoral Associate, Infectious Disease and Microbiome Program at The Broad Institute of MIT and Harvard, 2018
Ph.D. Computer Science and minors in Bioinformatics and Applied Statistics, New Mexico State University, 2014
M.S. Computer Science and minor in Bioinformatics, New Mexico State University, 2013
M.S. Computer Engineering, Shiraz University, 2005
B.S. Computer Engineering, Razi University of Kermanshah, 2003
EXPERTISE:
Metabolomics
Metagenomics
Computational Biology and Bioinformatics
Biostatistics
Omics Data Science
TEACHING:
PUBH 8885 - Computational Biology
PUBH 6859 - High Performance and Cloud Computing
PUBH 3242 - PUBH 6899 Health Data Visualization
PUBH 6897 - Research in Bioststatsic and Bioinformatics
INSTITUTES AND CENTERS:
Department of Biostatistics and Bioinformatics
Computational Biology Institute
District of Columbia Center for AIDS Research (DC FAR)
GW Cancer Center
GW Trustworthy AI Initiative
RESEARCH:
The theme of the Rahnavard Lab is Omics Data Science for Public Health and Precision Medicine. Advances in high-throughput technologies enable capturing snapshots of human biology. These technologies include DNA sequencing techniques, liquid chromatography-mass spectrometry (LC-MS), single-cell RNA sequencing (scRNA-seq), and magnetic resonance angiogram (MRA), which are ideal tools for jointly characterizing the host-biomes interactions.
Rahnavard Lab develops innovative computational, machine learning, deep learning, and statistical methods aimed at achieving actionable research outcomes in health and disease using high-dimensional omics data. The goals for these techniques are to provide approaches to find biological patterns in the zoomed-in personalized level and the zoomed-out population-level using omics data.
A key challenge of using omics data is the noise introduced by machines and batch effects which suppress the biological signals. We develop computational and deep learning methods to process and correct metabolite profiles. Clean data is fundamental to finding patterns and associations in high-dimensional data. Curing metabolomics data enable the translation of microbiome-metabolite discoveries into treatments for human disease, a key focus of the research laboratory. Profiling the human microbiome and the human metabolome in the context of health and disease is critical for translational biomedical research.
The lab also applies computational methods to multi-omics data to generate hypotheses of the underlying processes involved in disease activity. The Rahnavard lab also tests hypotheses with strong evidence in measured data in a laboratory and translation into actionable diagnostics and therapeutics.
1. Harriett Fuller, Yiwen Zhu, Jayna Nicholas, Haley A. Chatelaine, Emily M. Drzymalla, Afrand K. Sarvestani, Sachelly Julián-Serrano, Nasa Sinnott-Armstrong, Laura M. Raffield, Ali Rahnavard, Katherine H. Shutta, Xinwei Hua, Burcu F. Darst, The Emerging Field of Metabolomic Epidemiology Offers Novel Biological Insights into Disease Etiology, in press, Nature Metabolism
2. Rahnavard A, Chatterjee R, Wen H, Gaylord C, Mugusi S, Klatt KC, Smith ER. Molecular epidemiology of pregnancy using omics data: advances, success stories, and challenges. available at Research Square [https://doi.org/10.21203/rs.3.rs-2214567/v1]
3. Mahdi Baghbanzadeh, Tyson Dawson, Bahar Sayoldin et al. deepBreaks: a Machine Learning Tool for Identifying and Prioritizing Genotype-phenotype Associations, 01 February 2023, PREPRINT (Version 1) available at Research Square [https://doi.org/10.21203/rs.3.rs-2534899/v1]
4. Joshi AD, Rahnavard A, Kachroo P, Mendez KM, Lawrence W, Julián-Serrano S, Hua X, Fuller H, Sinnott-Armstrong N, Tabung FK, Shutta KH, Raffield LM, Darst BF. An epidemiological introduction to human metabolomic investigations. Trends Endocrinol Metab. 2023 Sep;34(9):505-525. doi: 10.1016/j.tem.2023.06.006. Epub 2023 Jul 17. Review. PubMed PMID: 37468430.
5. Rahnavard, A. Omics correlation for efficient network construction. Nature Computational Science 3, 285–286 (2023). https://doi.org/10.1038/s43588-023-00436-z
6. Bustamante JM, Dawson T, Loeffler C, Marfori Z, Marchesi JR, Mullish BH, Thompson CC, Crandall KA, Rahnavard A, Allegretti JR, Cummings BP. Impact of Fecal Microbiota Transplantation on Gut Bacterial Bile Acid Metabolism in Humans. Nutrients. 2022 Dec 7;14(24). doi: 10.3390/nu14245200. PubMed PMID: 36558359; PubMed Central PMCID: PMC9785599.
7. Mallick H, Chatterjee S, Chowdhury S, Chatterjee S, Rahnavard A, Hicks SC. Differential expression of single-cell RNA-seq data using Tweedie models. Stat Med. 2022 Aug 15;41(18):3492-3510. doi: 10.1002/sim.9430. Epub 2022 Jun 2. PubMed PMID: 35656596; PubMed Central PMCID: PMC9288986.
8. Rahnavard A, Mann B, Giri A, Chatterjee R, Crandall KA. Metabolite, protein, and tissue dysfunction associated with COVID-19 disease severity. Sci Rep. 2022 Jul 16;12(1):12204. doi: 10.1038/s41598-022-16396-9. PubMed PMID: 35842456; PubMed Central PMCID: PMC9288092.
9. Ghazi AR, Sucipto K, Rahnavard A, Franzosa EA, McIver LJ, Lloyd-Price J, Schwager E, Weingart G, Moon YS, Morgan XC, Waldron L, Huttenhower C. High-sensitivity pattern discovery in large, paired multiomic datasets. Bioinformatics. 2022 Jun 24;38(Suppl 1):i378-i385. doi: 10.1093/bioinformatics/btac232. PubMed PMID: 35758795; PubMed Central PMCID: PMC9235493.
10. Zhang Y, Bhosle A, Bae S, McIver LJ, Pishchany G, Accorsi EK, Thompson KN, Arze C, Wang Y, Subramanian A, Kearney SM, Pawluk A, Plichta DR, Rahnavard A, Shafquat A, Xavier RJ, Vlamakis H, Garrett WS, Krueger A, Huttenhower C, Franzosa EA. Discovery of bioactive microbial gene products in inflammatory bowel disease. Nature. 2022 Jun;606(7915):754-760. doi: 10.1038/s41586-022-04648-7. Epub 2022 May 25. PubMed PMID: 35614211; PubMed Central PMCID: PMC9913614.
11. Chow JH, Rahnavard A, Gomberg-Maitland M, Chatterjee R, Patodi P, Yamane DP, Levine AR, Davison D, Hawkins K, Jackson AM, Quintana MT, Lankford AS, Keneally RJ, Al-Mashat M, Fisher D, Williams J, Berger JS, Mazzeffi MA, Crandall KA. Association of Early Aspirin Use With In-Hospital Mortality in Patients With Moderate COVID-19. JAMA Netw Open. 2022 Mar 1;5(3):e223890. doi: 10.1001/jamanetworkopen.2022.3890. PubMed PMID: 35323950; PubMed Central PMCID: PMC8948531.
12. Rahnavard A, Dawson T, Clement R, Stearrett N, Pérez-Losada M, Crandall KA. Epidemiological associations with genomic variation in SARS-CoV-2. Sci Rep. 2021 Nov 26;11(1):23023. doi: 10.1038/s41598-021-02548-w. PubMed PMID: 34837008; PubMed Central PMCID: PMC8626494.
13. Mallick H, Rahnavard A, McIver LJ, Ma S, Zhang Y, Nguyen LH, Tickle TL, Weingart G, Ren B, Schwager EH, Chatterjee S, Thompson KN, Wilkinson JE, Subramanian A, Lu Y, Waldron L, Paulson JN, Franzosa EA, Bravo HC, Huttenhower C. Multivariable association discovery in population-scale meta-omics studies. PLoS Comput Biol. 2021 Nov;17(11):e1009442. doi: 10.1371/journal.pcbi.1009442. eCollection 2021 Nov. PubMed PMID: 34784344; PubMed Central PMCID: PMC8714082.
14. Rahnavard A, Chatterjee S, Sayoldin B, Crandall KA, Tekola-Ayele F, Mallick H. Omics community detection using multi-resolution clustering. Bioinformatics. 2021 Oct 25;37(20):3588-3594. doi: 10.1093/bioinformatics/btab317. PubMed PMID: 33974004; PubMed Central PMCID: PMC8545346.
15. Smith ER, He S, Klatt KC, Barberio MD, Rahnavard A, Azad N, Brandt C, Harker B, Hogan E, Kucherlapaty P, Moradian D, Gernand AD, Ahmadzia HK. Limited data exist to inform our basic understanding of micronutrient requirements in pregnancy. Sci Adv. 2021 Oct 22;7(43):eabj8016. doi: 10.1126/sciadv.abj8016. Epub 2021 Oct 22. PubMed PMID: 34678054; PubMed Central PMCID: PMC8535830.
16. Bennett TD, Moffitt RA, Hajagos JG, Amor B, Anand A, Bissell MM, Bradwell KR, Bremer C, Byrd JB, Denham A, DeWitt PE, Gabriel D, Garibaldi BT, Girvin AT, Guinney J, Hill EL, Hong SS, Jimenez H, Kavuluru R, Kostka K, Lehmann HP, Levitt E, Mallipattu SK, Manna A, McMurry JA, Morris M, Muschelli J, Neumann AJ, Palchuk MB, Pfaff ER, Qian Z, Qureshi N, Russell S, Spratt H, Walden A, Williams AE, Wooldridge JT, Yoo YJ, Zhang XT, Zhu RL, Austin CP, Saltz JH, Gersing KR, Haendel MA, Chute CG. Clinical Characterization and Prediction of Clinical Severity of SARS-CoV-2 Infection Among US Adults Using Data From the US National COVID Cohort Collaborative. JAMA Netw Open. 2021 Jul 1;4(7):e2116901. doi: 10.1001/jamanetworkopen.2021.16901. PubMed PMID: 34255046; PubMed Central PMCID: PMC8278272.
17. Amritphale A, Chatterjee R, Chatterjee S, Amritphale N, Rahnavard A, Awan GM, Omar B, Fonarow GC. Predictors of 30-Day Unplanned Readmission After Carotid Artery Stenting Using Artificial Intelligence. Adv Ther. 2021 Jun;38(6):2954-2972. doi: 10.1007/s12325-021-01709-7. Epub 2021 Apr 9. PubMed PMID: 33834355; PubMed Central PMCID: PMC8190015.
18. Nikookar E, Naderi E, Rahnavard A. Cervical Cancer Prediction by Merging Features of Different Colposcopic Images and Using Ensemble Classifier. J Med Signals Sens. 2021 Apr-Jun;11(2):67-78. doi: 10.4103/jmss.JMSS_16_20. eCollection 2021 Apr-Jun. PubMed PMID: 34268095; PubMed Central PMCID: PMC8253312.
19. Stearrett N, Dawson T, Rahnavard A, Bachali P, Bendall ML, Zeng C, Caricchio R, Pérez-Losada M, Grammer AC, Lipsky PE, Crandall KA. Expression of Human Endogenous Retroviruses in Systemic Lupus Erythematosus: Multiomic Integration With Gene Expression. Front Immunol. 2021;12:661437. doi: 10.3389/fimmu.2021.661437. eCollection 2021. PubMed PMID: 33986751; PubMed Central PMCID: PMC8112243.
20. Haendel MA, Chute CG, Bennett TD, Eichmann DA, Guinney J, Kibbe WA, Payne PRO, Pfaff ER, Robinson PN, Saltz JH, Spratt H, Suver C, Wilbanks J, Wilcox AB, Williams AE, Wu C, Blacketer C, Bradford RL, Cimino JJ, Clark M, Colmenares EW, Francis PA, Gabriel D, Graves A, Hemadri R, Hong SS, Hripscak G, Jiao D, Klann JG, Kostka K, Lee AM, Lehmann HP, Lingrey L, Miller RT, Morris M, Murphy SN, Natarajan K, Palchuk MB, Sheikh U, Solbrig H, Visweswaran S, Walden A, Walters KM, Weber GM, Zhang XT, Zhu RL, Amor B, Girvin AT, Manna A, Qureshi N, Kurilla MG, Michael SG, Portilla LM, Rutter JL, Austin CP, Gersing KR. The National COVID Cohort Collaborative (N3C): Rationale, design, infrastructure, and deployment. J Am Med Inform Assoc. 2021 Mar 1;28(3):427-443. doi: 10.1093/jamia/ocaa196. PubMed PMID: 32805036; PubMed Central PMCID: PMC7454687.
21. He S, Klatt KC, Rahnavard A, Barberio MD, Gernand AD, Smith ER. Protocol for meta-research on the evidence informing micronutrient dietary reference intakes for pregnant and lactating women. Gates Open Res. 2020;4:171. doi: 10.12688/gatesopenres.13199.1. eCollection 2020. PubMed PMID: 33629039; PubMed Central PMCID: PMC7876347.
22. Thingholm LB, Rühlemann MC, Koch M, Fuqua B, Laucke G, Boehm R, Bang C, Franzosa EA, Hübenthal M, Rahnavard A, Frost F, Lloyd-Price J, Schirmer M, Lusis AJ, Vulpe CD, Lerch MM, Homuth G, Kacprowski T, Schmidt CO, Nöthlings U, Karlsen TH, Lieb W, Laudes M, Franke A, Huttenhower C. Obese Individuals with and without Type 2 Diabetes Show Different Gut Microbial Functional Capacity and Composition. Cell Host Microbe. 2019 Aug 14;26(2):252-264.e10. doi: 10.1016/j.chom.2019.07.004. Epub 2019 Aug 6. PubMed PMID: 31399369; PubMed Central PMCID: PMC7720933.
23. Lloyd-Price J, Arze C, Ananthakrishnan AN, Schirmer M, Avila-Pacheco J, Poon TW, Andrews E, Ajami NJ, Bonham KS, Brislawn CJ, Casero D, Courtney H, Gonzalez A, Graeber TG, Hall AB, Lake K, Landers CJ, Mallick H, Plichta DR, Prasad M, Rahnavard G, Sauk J, Shungin D, Vázquez-Baeza Y, White RA 3rd, Braun J, Denson LA, Jansson JK, Knight R, Kugathasan S, McGovern DPB, Petrosino JF, Stappenbeck TS, Winter HS, Clish CB, Franzosa EA, Vlamakis H, Xavier RJ, Huttenhower C. Multi-omics of the gut microbial ecosystem in inflammatory bowel diseases. Nature. 2019 May;569(7758):655-662. doi: 10.1038/s41586-019-1237-9. Epub 2019 May 29. Review. PubMed PMID: 31142855; PubMed Central PMCID: PMC6650278.
24. Franzosa EA, McIver LJ, Rahnavard G, Thompson LR, Schirmer M, Weingart G, Lipson KS, Knight R, Caporaso JG, Segata N, Huttenhower C. Species-level functional profiling of metagenomes and metatranscriptomes. Nat Methods. 2018 Nov;15(11):962-968. doi: 10.1038/s41592-018-0176-y. Epub 2018 Oct 30. PubMed PMID: 30377376; PubMed Central PMCID: PMC6235447.
25. McDonald D, Hyde E, Debelius JW, Morton JT, Gonzalez A, Ackermann G, Aksenov AA, Behsaz B, Brennan C, Chen Y, DeRight Goldasich L, Dorrestein PC, Dunn RR, Fahimipour AK, Gaffney J, Gilbert JA, Gogul G, Green JL, Hugenholtz P, Humphrey G, Huttenhower C, Jackson MA, Janssen S, Jeste DV, Jiang L, Kelley ST, Knights D, Kosciolek T, Ladau J, Leach J, Marotz C, Meleshko D, Melnik AV, Metcalf JL, Mohimani H, Montassier E, Navas-Molina J, Nguyen TT, Peddada S, Pevzner P, Pollard KS, Rahnavard G, Robbins-Pianka A, Sangwan N, Shorenstein J, Smarr L, Song SJ, Spector T, Swafford AD, Thackray VG, Thompson LR, Tripathi A, Vázquez-Baeza Y, Vrbanac A, Wischmeyer P, Wolfe E, Zhu Q, Knight R. American Gut: an Open Platform for Citizen Science Microbiome Research. mSystems. 2018 May-Jun;3(3). doi: 10.1128/mSystems.00031-18. eCollection 2018 May-Jun. PubMed PMID: 29795809; PubMed Central PMCID: PMC5954204.
26. Kolde R, Franzosa EA, Rahnavard G, Hall AB, Vlamakis H, Stevens C, Daly MJ, Xavier RJ, Huttenhower C. Host genetic variation and its microbiome interactions within the Human Microbiome Project. Genome Med. 2018 Jan 29;10(1):6. doi: 10.1186/s13073-018-0515-8. PubMed PMID: 29378630; PubMed Central PMCID: PMC5789541.
27. Lloyd-Price J, Mahurkar A, Rahnavard G, Crabtree J, Orvis J, Hall AB, Brady A, Creasy HH, McCracken C, Giglio MG, McDonald D, Franzosa EA, Knight R, White O, Huttenhower C. Erratum: Strains, functions and dynamics in the expanded Human Microbiome Project. Nature. 2017 Nov 9;551(7679):256. doi: 10.1038/nature24485. Epub 2017 Oct 12. PubMed PMID: 29022944; PubMed Central PMCID: PMC7608344.
28. Lloyd-Price J, Mahurkar A, Rahnavard G, Crabtree J, Orvis J, Hall AB, Brady A, Creasy HH, McCracken C, Giglio MG, McDonald D, Franzosa EA, Knight R, White O, Huttenhower C. Strains, functions and dynamics in the expanded Human Microbiome Project. Nature. 2017 Oct 5;550(7674):61-66. doi: 10.1038/nature23889. Epub 2017 Sep 20. PubMed PMID: 28953883; PubMed Central PMCID: PMC5831082.
29. Börnigen D, Moon YS, Rahnavard G, Waldron L, McIver L, Shafquat A, Franzosa EA, Miropolsky L, Sweeney C, Morgan XC, Garrett WS, Huttenhower C. A reproducible approach to high-throughput biological data acquisition and integration. PeerJ. 2015;3:e791. doi: 10.7717/peerj.791. eCollection 2015. PubMed PMID: 26157642; PubMed Central PMCID: PMC4493686.